Correlations
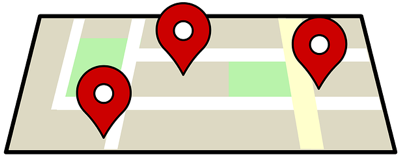
What can we use to predict the value of a home? Some factors are easily quantifiable, such as number of bedrooms and square feet, while others are hard to find and harder to quantify, such as the amount of sunlight that illuminates the kitchen on a Saturday morning.
Using R, a statistical programming language, I looked at 48,526 records of single-family houses and 19,453 condominiums sold mostly in Massachusetts over the past twelve months, and checked correlations1 between the sale price and various data points available on their listing sheets.
The property’s location, measured by zip code, had the single strongest relationship to the final sale price. This gives credence to our mantra “Location Location Location!”
Zip code | Bathrooms | Rooms | Square feet | Bedrooms | Garage spaces | Central air? | Lot size | Lender owned? |
0.73 | 0.63 | 0.54 | 0.52 | 0.45 | 0.32 | 0.31 | 0.02 | -0.13 |
(Significance: p-value < 2.2e-16).
Limiting to just parts of the Boston area2, the strongest relationships are number of bathrooms and zip code. Garage spaces and lot size jump in importance, highlighting how those are not taken for granted in this area. Surprisingly, the age of the house did not have a linear relationship to the sale price.
Bathrooms | Zip code | Rooms | Square feet | Bedrooms | Garage spaces | Central air? | Lot size | Age | Lender owned? |
0.73 | 0.72 | 0.63 | 0.51 | 0.51 | 0.42 | 0.35 | 0.31 | 0.0 | -0.07 |
(Significance: p-value < 2.2e-16, except for age p-value = 0.8528, and lender owned p-value = 9.395e-05).
Okay, so can we use these to help predict the value of a particular house? Using the strongest factors, I was able to build (with help from my sister, thanks Elana!) a linear regression model which predicts 86% of the variability in price. (R-squared = 0.862)
Only 86%? That’s actually not bad as far as these things go, but it does leave a lot of unaccounted for variability in the sale prices. That’s where those harder to quantify factors come in. Was the kitchen updated? Is a bidding war expected? Is there a lot of clutter? Are the sellers in a rush? Why is there a replica of the Manneken-Pis statue in the backyard? (-0.45…just kidding)
This is why site unseen estimates that you find online should be considered only as starting points. An experienced REALTOR® will consider a range of factors such as these and may consult multiple models when advising you on the the value of your home, or a fair offer price for a property you wish to buy. Email me at avi@avirealestate.com to learn more.
1. What do those correlation numbers mean? A correlation coefficient measures the statistical relationship between two variables and runs from +1 to -1. A positive value means that as one variable (eg. square feet) increases, so does the other variable (eg. price). A negative value means that as one variable increases (eg. crime rate), the other variable (eg. sale price) decreases. A value of 0 means that there is no linear relationship (eg. house color and sale price.)
2. Boston, Brookline, Cambridge, Somerville, Medford, Newton, Waltham, Watertown, Natick, and Wellesley.
3. Data set includes 46,808 records from Massachusetts, 896 from New Hampshire, 664 from Rhode Island, 142 from Connecticut, and 2 from Maine, for single-family homes sold in the past twelve months.
Coming soon: Using these statistical models, Phil Ganz (a top-rated Boston-based mortgage broker) and I are developing an innovative search tool to help prospective buyers identify homes. It’s still under development, but I’ll let you know when it’s ready for testing!
Previous reports: In previous reports we’ve discussed market performance in 2015, seasonality, and price-to-rent ratios.
Questions? Email me and let's set up a time to discuss your real estate needs.
AVI KAUFMAN is a top broker who lives in Brookline, Massachusetts and works there and surrounding communities, assisting buyers and sellers of residential property. He is building a unique practice dedicated to serving the best interest of his clients - see how he's different.